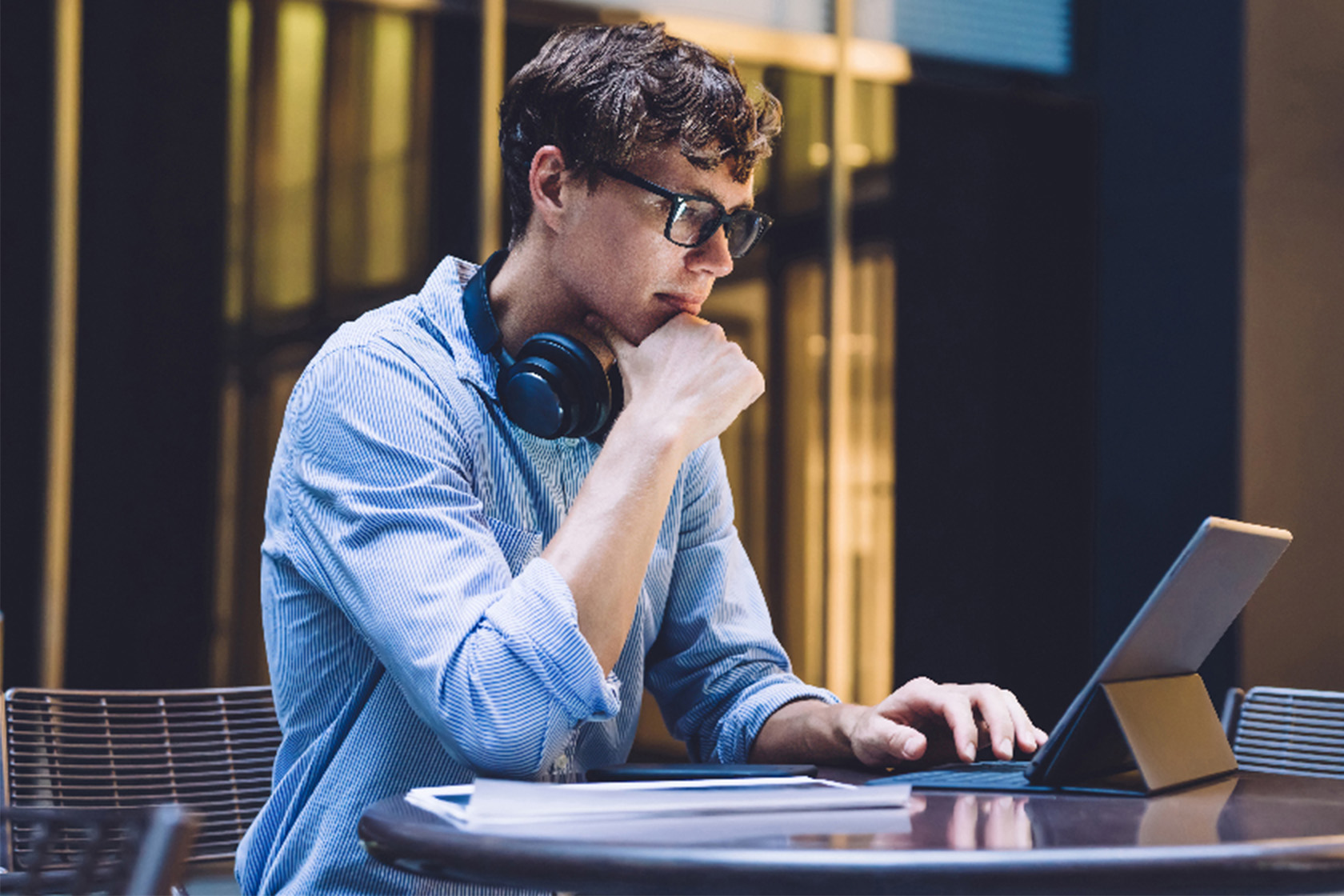
In the rapidly evolving technology landscape, large Language Models (LLMs) have emerged as a leading innovation, transforming the way we interact with technology and opening doors to new possibilities.
Training Deep Learning-based Language Models to have competent conversations with artificial intelligence is an exciting challenge that involves a combination of supervised learning techniques, reinforcement, and contextual adaptation.
Virtual Assistants powered by Deep Learning-based Language Models (LLM) have transformed the way we interact with technology.
In this article, we will explore 8 key strategies for training LLMs and building virtual assistants capable of delivering exceptional experiences.
8 Key Strategies for Effectively Training LLMs
- Conversation Data Training
The first step in improving an LLM’s conversational skills is to provide him or her with a large amount of conversational data. This includes dialogues and discussions covering a variety of topics and interaction styles. This data should cover a range of topics and situations so that the assistant can understand and respond to a wide range of queries.
- Reinforcement Learning
Supervised training is essential for the attendant to learn to generate consistent and accurate responses. However, combining it with reinforcement learning can take it to a higher level. Through user feedback and evaluation of the quality of responses, the assistant can learn to improve its responses based on experience.
- Advanced Context Modeling
Understanding context is critical in a meaningful conversation. LLMs must be trained to understand not only the current query, but also the context of the previous conversation. This ensures that responses are relevant and consistent throughout the interaction.
- Real-time user feedback
User feedback is a valuable source of improvement for virtual assistants. Providing an easy way for users to rate and give feedback on the assistant’s responses can help to continuously adjust the model and improve its capabilities. These evaluations can be used as feedback signals to refine the model during the training process.
- Generating creative responses
A powerful virtual assistant not only provides accurate, but also creative and natural responses. LLMs must be trained to generate responses that do not sound robotic but reflect how a human being might respond in a similar situation. Models must learn to avoid offensive, inappropriate or misleading responses.
- Adaptation to Individual Users
Users have unique conversational styles and preferences. Advanced LLMs can be trained to adapt to a specific user over time. This can be accomplished by allowing the model to interact with the same user multiple times and learn from their choices and feedback.
- Multilingual and Cultural Integration
A powerful virtual assistant must be able to understand and respond in multiple languages and cultural contexts. Training in multilingual data and diverse cultural expressions is essential to achieve this capability.
- Rigorous Testing and Continuous Optimization
Once the virtual assistant has been implemented, rigorous testing is crucial. Potential problems must be identified and addressed, erroneous responses corrected, and the model adjusted based on actual usage results.
eva: orchestrating LLMs
LLMs have proven to be a milestone in AI and their journey promises exciting and transformative terrain. Their future is characterized by constant improvement, increased personalization, real-world applications, and a pivotal role in creativity and education.
As these models become more conversationally proficient, they will be able to play a more integral role in a variety of applications, from advanced virtual assistants to customer support systems and beyond.
At NTT DATA, we go together with technological innovation, which is why our eva platform has created an orchestrator for LLMs that simplifies complex interactions.
This new functionality enhances our platform’s ability to orchestrate calls to orchestrate calls to generative AI tools, such as Azure OpenAI services, making it easier to handle more advanced and complex tasks with unprecedented simplicity and elegance.
At eva, we use a variety of generative AI models provided by Azure OpenAI (and other vendors) to meet various needs, such as content generation, classification, and data processing.
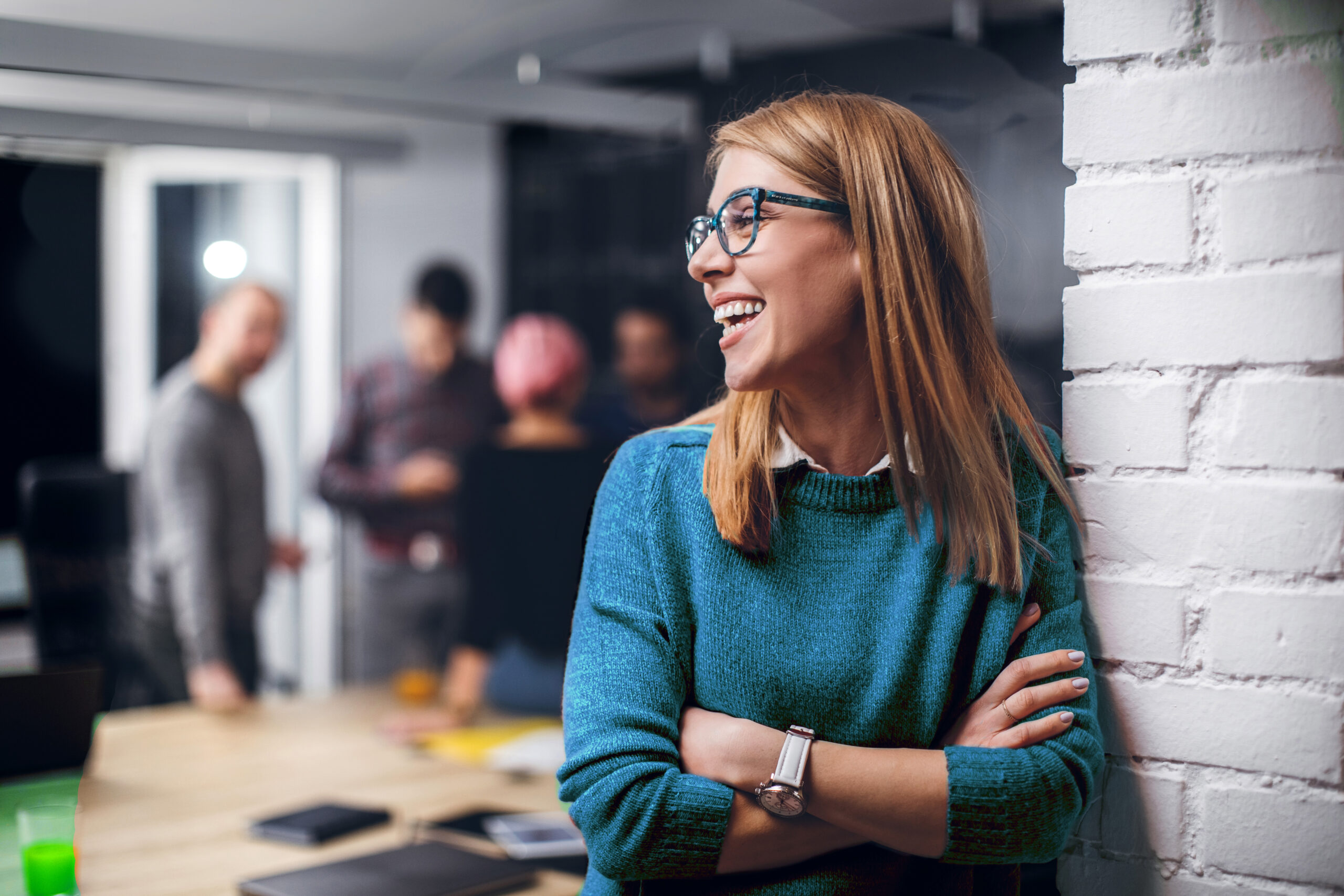
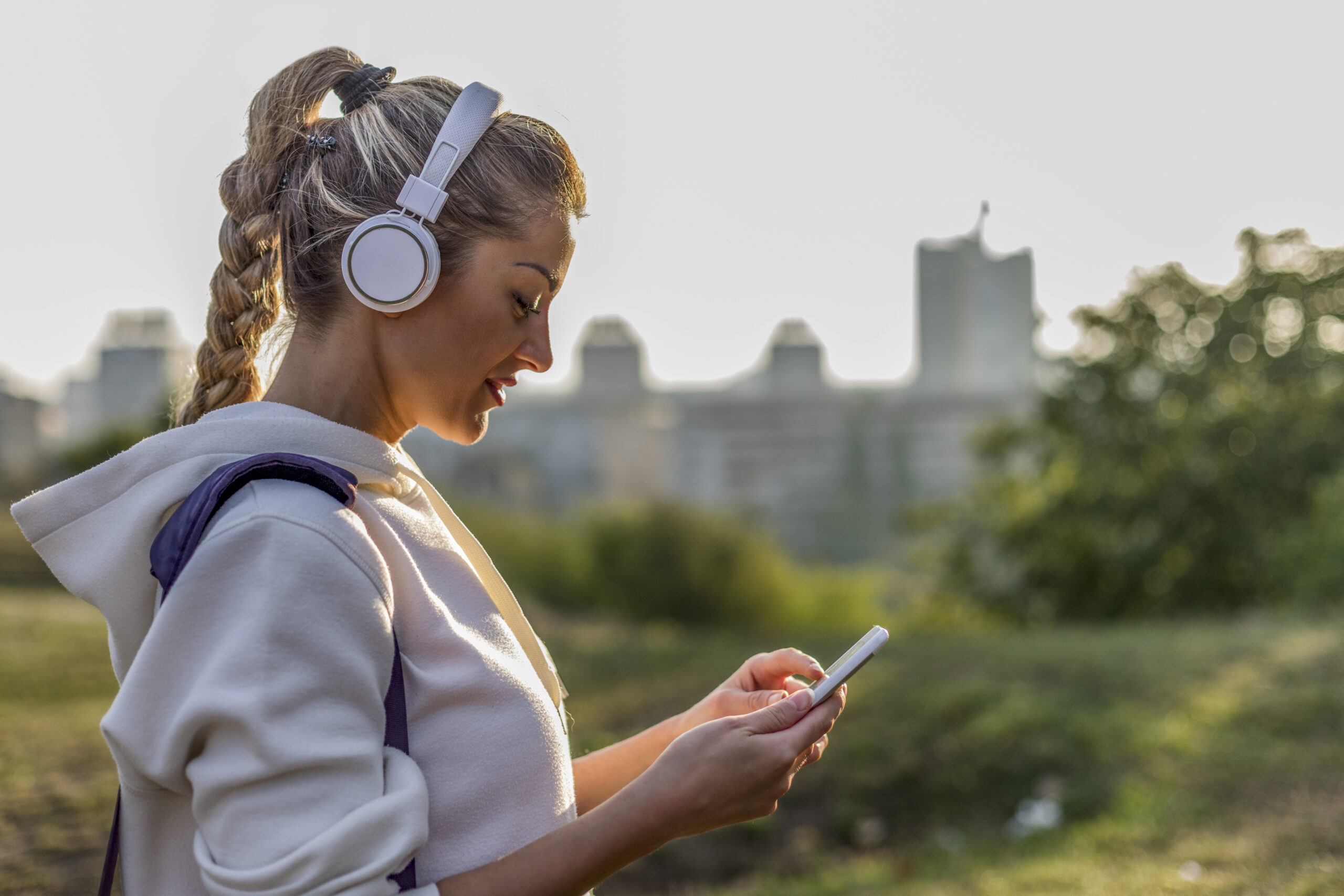
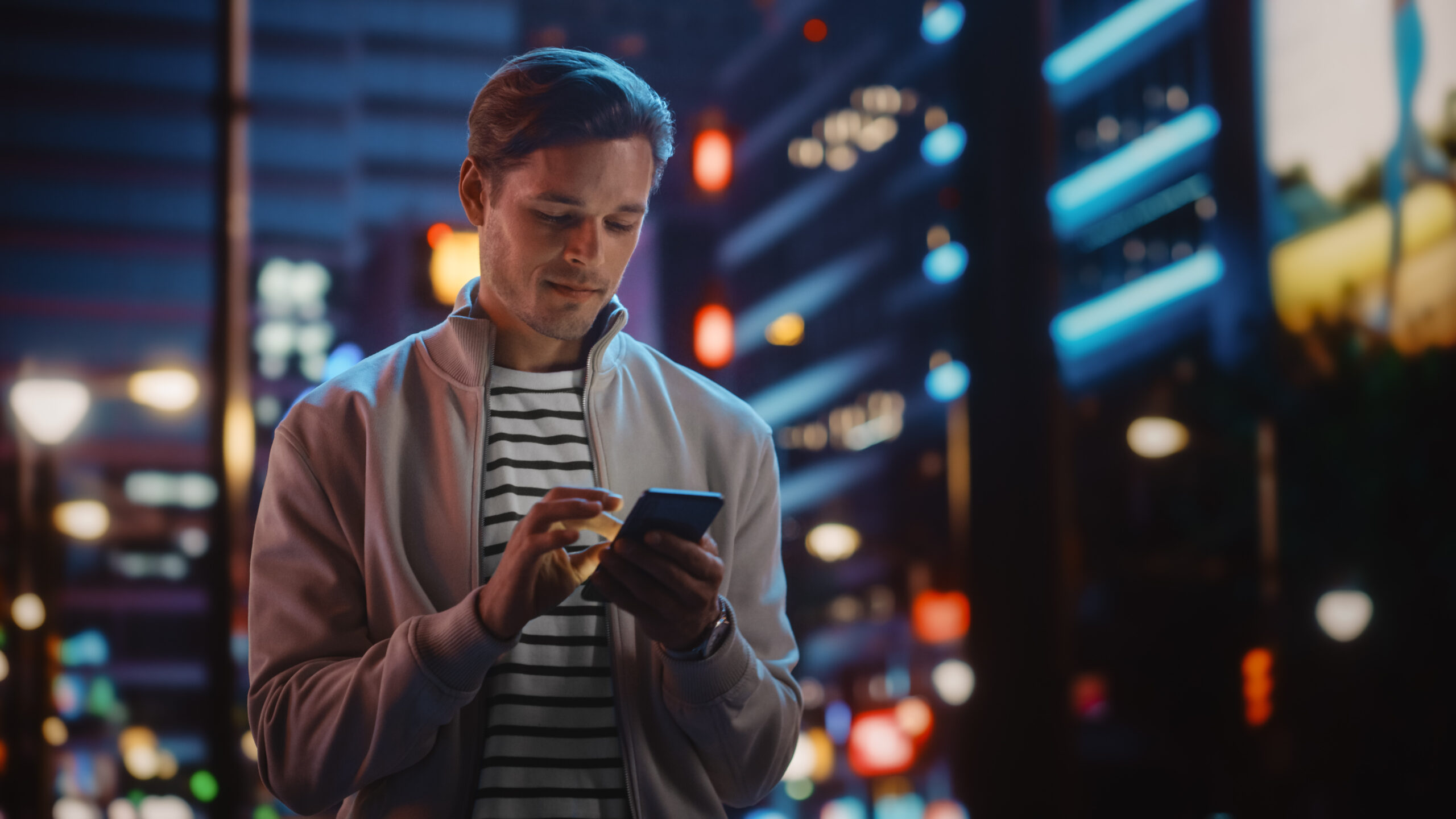
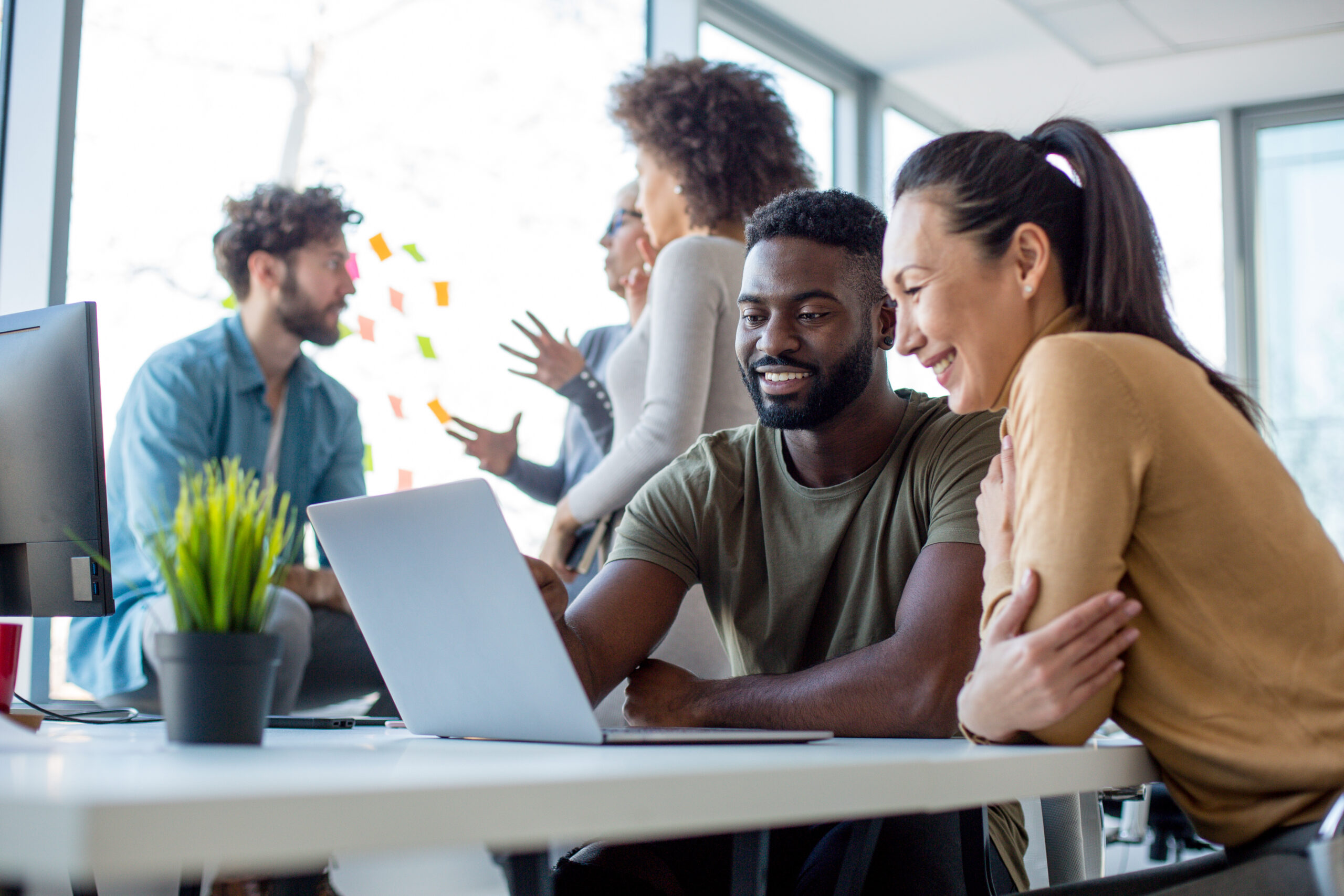